Reducing unnecessary expenses is something that any successful business needs to focus on so that those funds can be redirected towards more fruitful pursuits. With the prevailing economic conditions, trimming waste is perhaps more important now than it has been in decades. All this talk of belt-tightening may sound difficult or painful, but in reality, most companies have a lot of low-hanging fruit that can be dealt with pretty easily, and yet have a large impact on the bottom line. One area in particular that is often neglected is unnecessary spending on energy consumption.
The U.S. Environmental Protection Agency estimates that a mind-boggling 30% of energy consumed by a typical commercial building is wasted. Much of this waste stems from heating, cooling, and illuminating unoccupied spaces. The engineering team over at Zalmotek saw this as an opportunity to save money and conserve energy, so they got to work designing a simple device to make buildings smarter and more efficient. They demonstrated a quick, easy solution to monitor the occupancy of a room with a little help from Edge Impulse’s machine learning development platform. That occupancy information could be used to turn off utilities where they are not needed.
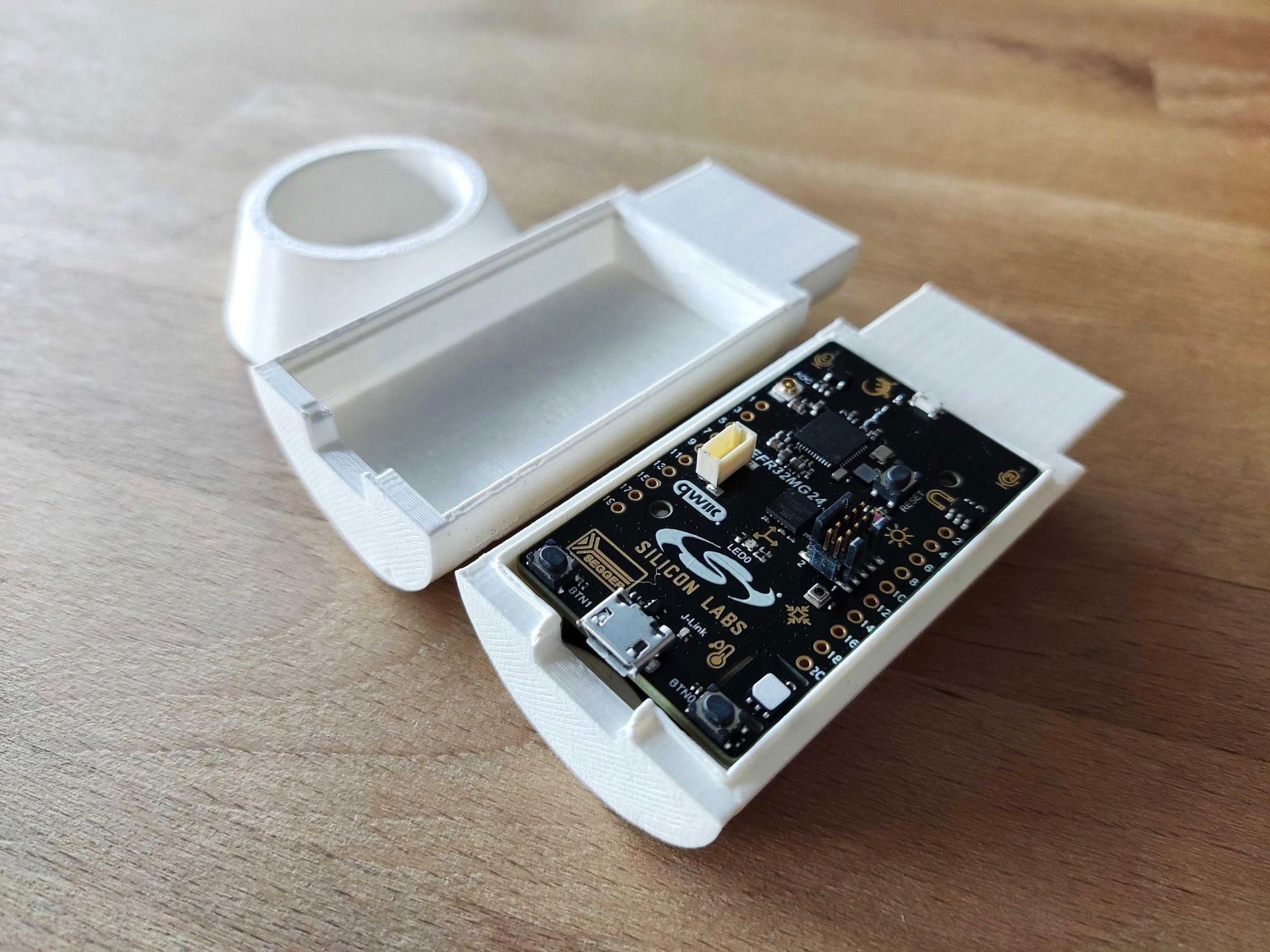
After considering several options, Zalmotek settled on a plan to classify audio samples with a neural network to determine room occupancy status. With Silicon Labs’ recent launch of the new EFR32MG24 wireless SoCs, they had the perfect platform for their device. This board has an Arm Cortex-M33 processor running at 78 MHz to power through machine learning algorithm inferences, and is loaded with sensors — including the microphone that Zalmotek requires, making it a simple, all-in-one solution. To keep the unit looking nice and protected from hazards like liquid spills, a 3D-printed case was also designed to house the development board. To make installation of a number of these devices around a building a breeze, the EFR32MG24 can be powered by a CR2030 coin cell battery.
There was not a lot of work involved in getting the hardware set up, so attention was quickly turned to training the neural network. A custom firmware image provided by Edge Impulse was downloaded and flashed to the board, which allowed it to connect directly to Edge Impulse Studio. With that connection in place, data could be collected from onboard sensors and automatically transferred into an Edge Impulse project. Zalmotek collected a very modest three minutes of audio samples for each class (occupied, unoccupied). The data acquisition tool automatically split this data between training and test datasets.
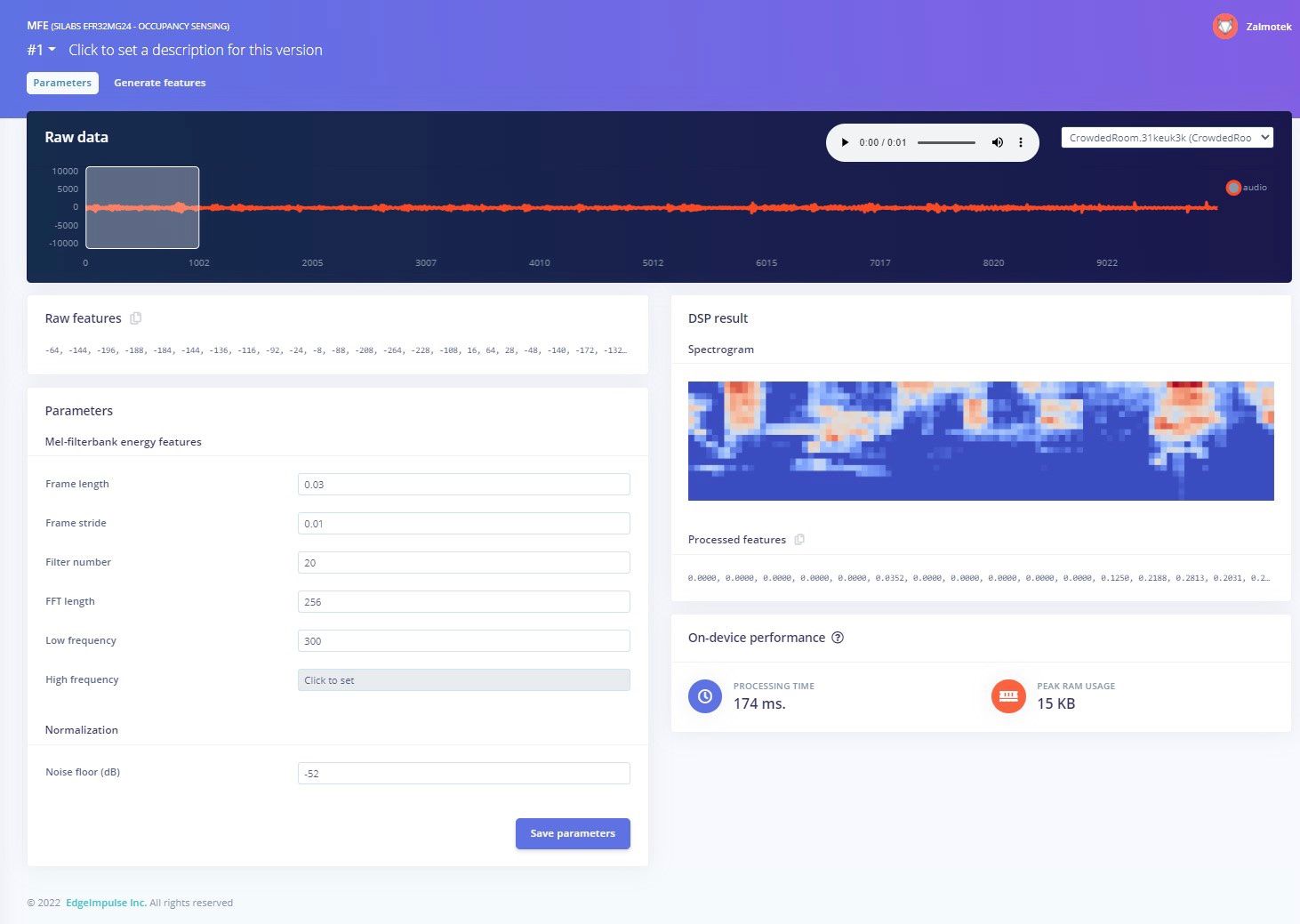
At this point, an impulse was created to process and classify the audio samples. Preprocessing steps were added to extract the most meaningful features from the data — this has the effect of producing more accurate classifications and also reducing the amount of computational resources that are required to run the algorithm. A neural network classifier was chosen to serve as the learning block for the impulse, after which the model training process was started. A training accuracy of 96.9% was observed — a quite impressive result from such a small training dataset. Moreover, the feature explorer showed a very clear separation between class clusters.
Despite the fantastic result, Zalmotek also wanted to see the model in action before deploying it to the hardware, so they used the live classification tool. This enabled them to perform an end to end test showing that data collected by the board’s sensors would be classified correctly. Confident in that testing, they downloaded a pre-built firmware image from Edge Impulse that contained the trained model, and everything that was needed to run it. By flashing that to the board, everything was up and running in an instant. Had they needed to add additional, custom functionality to the device, they could have also chosen to download the model as a self-contained C++ library that could be incorporated into a larger application with any necessary logic.
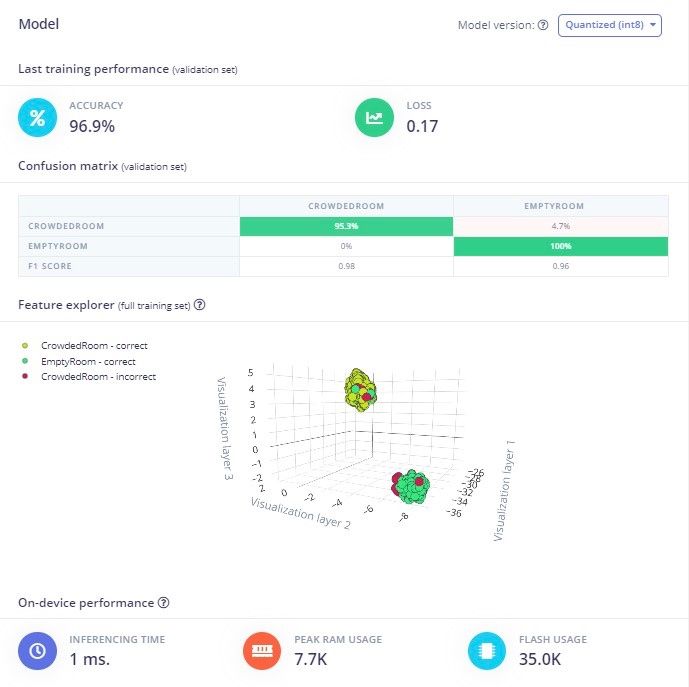
Zalmotek noted that between the sensor-packed Silicon Labs EFR32MG24 development board and the Edge Impulse platform, it was quick and easy to get this prototype up and running. Similar methods could be adapted to fit many other use cases as well, so be sure to read up on the project’s documentation for some great tips. And one final piece of advice — using Zalmotek’s public Edge Impulse project as a starting point is a great way to speed up your time to deployment.
Want to see Edge Impulse in action? Schedule a demo today.