With the rise of private spaceflight, there is a growing belief that traveling beyond the confines of planet Earth will soon become a commonplace experience. This is exciting news for the adventure seekers among us that dream of getting a glimpse of black sky firsthand, but it also means that getting nanosatellites into orbit will become easier and less expensive. And here at Edge Impulse, that gets us excited about AI at the edge — the edge of space, that is. Late last year, a step in this direction was taken when one of our favorite little development boards, the Sony Spresense, was sent into orbit in an effort to prove the utility of commercial, off-the-shelf computing platforms in space.
Engineer Dhruv Sheth is the type of person that gets very excited about developments such as these, and he wanted to get in on the action for himself. However, getting a satellite into orbit is still not within reach of the average person just yet. Sheth realized that, while he may not be able to get his device into space yet, he could still work with the same hardware that has been proven in a real nanosatellite. Using a Spresense board and Edge Impulse, he designed a device that can recognize the presence of clouds in images.
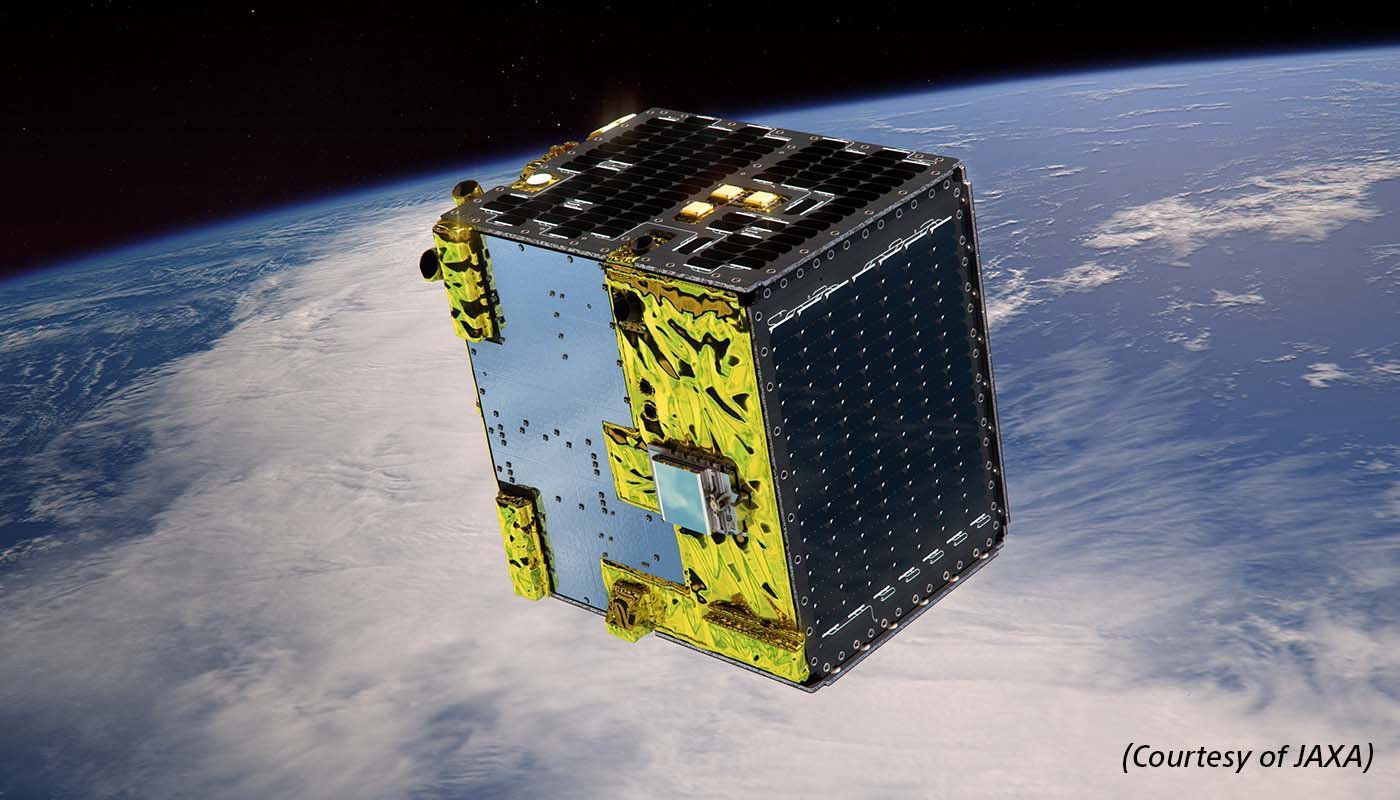
Satellite imagery has many applications in creating maps for navigation, locating mineral deposits, disaster recovery, and agricultural development. And this is where Sheth’s project comes into play — these images are of little value when clouds are blocking the view. With bandwidth being at a premium in space, transferring large, high-resolution images back to ground stations is not a good use of resources if the data is not informative. By determining if clouds are present in the images on-device, it is possible to discard useless data before transmitting it.
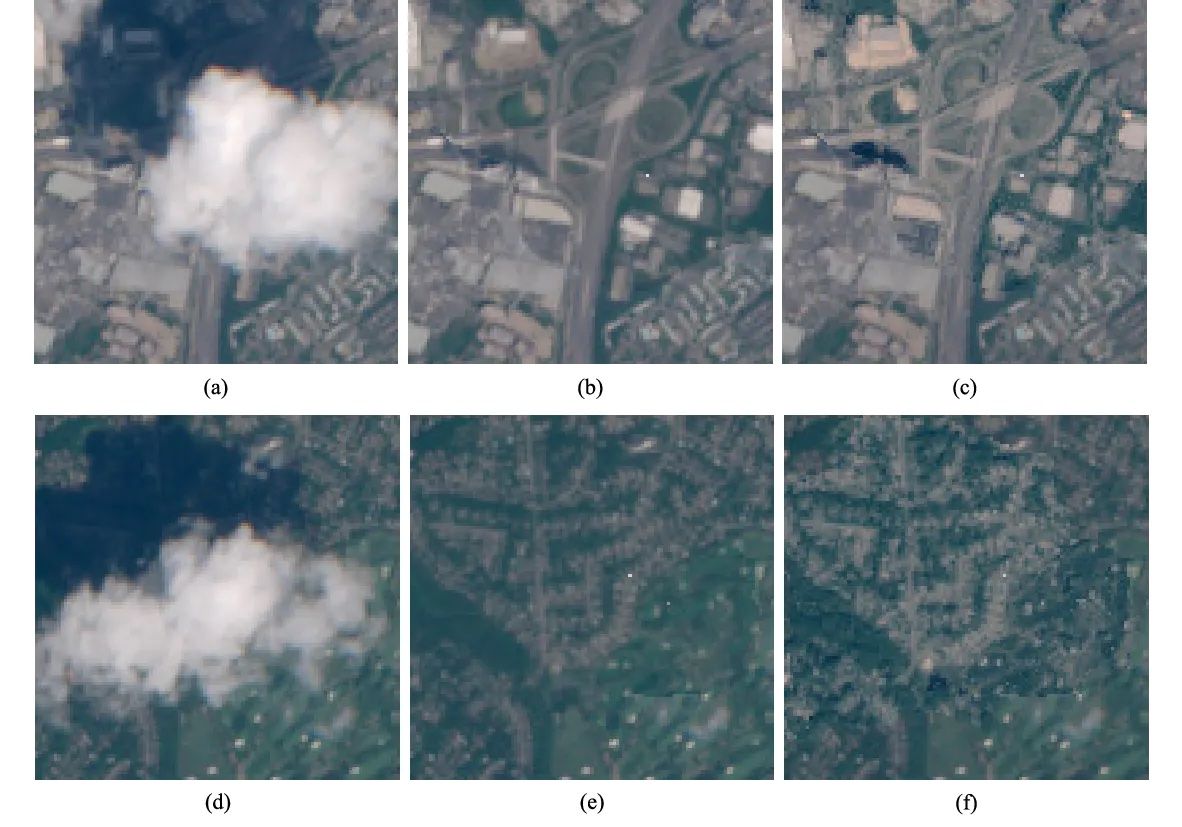
The Spresense is a powerful little board, with six Arm Cortex-M4F processor cores running at 156 MHz, 1.5 MB of SRAM, and 8 MB of flash memory. To put this compute power to work, a machine learning image classification pipeline was designed with the help of Edge Impulse. A dataset derived from images in NASA Worldview was downloaded from the Kaggle Cloud Classification Challenge as a first step. This data was then loaded into Edge Impulse with the data acquisition tool. 80% of the 810 images were selected for training the machine learning model, with the rest being held out to test the model’s accuracy later.
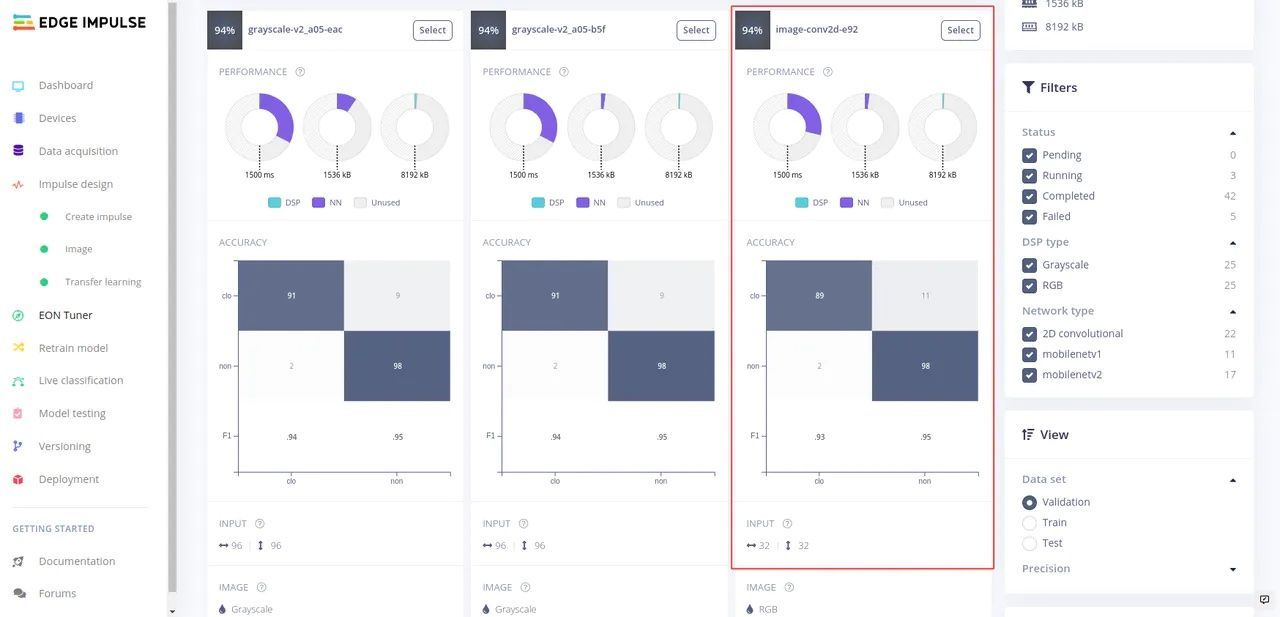
When working with embedded hardware, it is critical that a model’s parameters be optimally tuned to minimize computational complexity and memory requirements. Sheth used Edge Impulse’s EON Tuner to automatically choose the best model architecture and parameters for the task. The EON Tuner takes into account model accuracy and resource utilization to allow the user to choose the most appropriate model. Sheth found an option that was able to classify images with 94% accuracy, all while fitting comfortably within the constraints of the hardware.
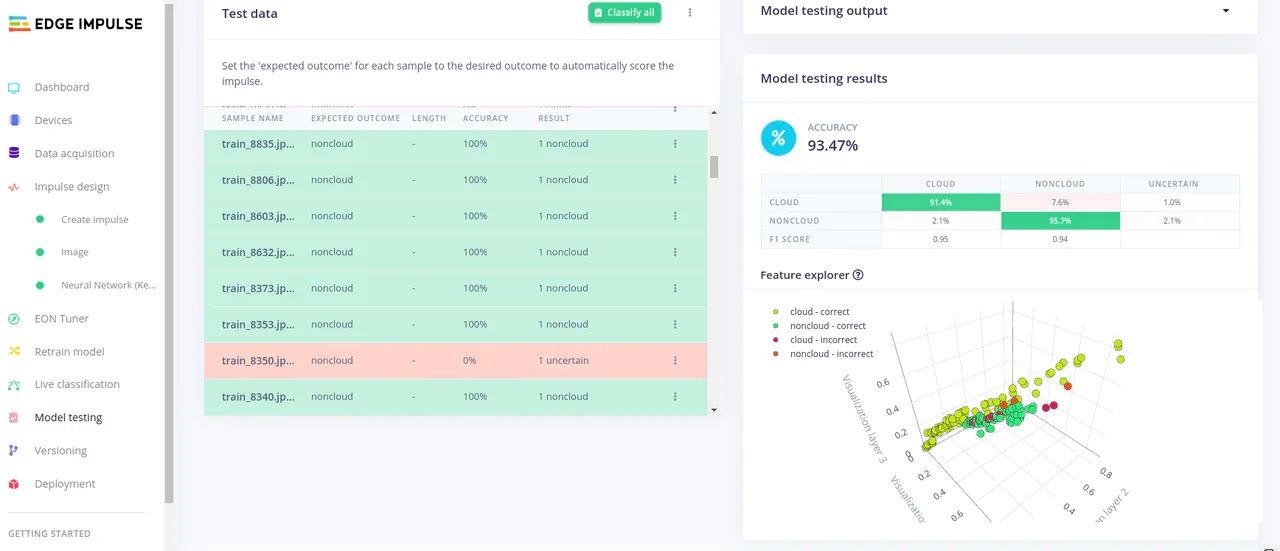
The Sony Spresense is fully compatible with Edge Impulse, so deploying the model was as simple as clicking a button on the “Deployment” tab. This generated a pre-compiled binary that can be flashed to the board. Sheth notes that this binary could even be deployed wirelessly to a Spresense that is orbiting the Earth in a nanosatellite.
Sheth is already exploring new ways that he can leverage Edge Impulse and the Sony Spresense to solve problems in space. One idea he had is to design a model that can classify different terrains, with the goal of minimizing the required image resolution and device power consumption to make satellite-based analyses more useful and efficient.
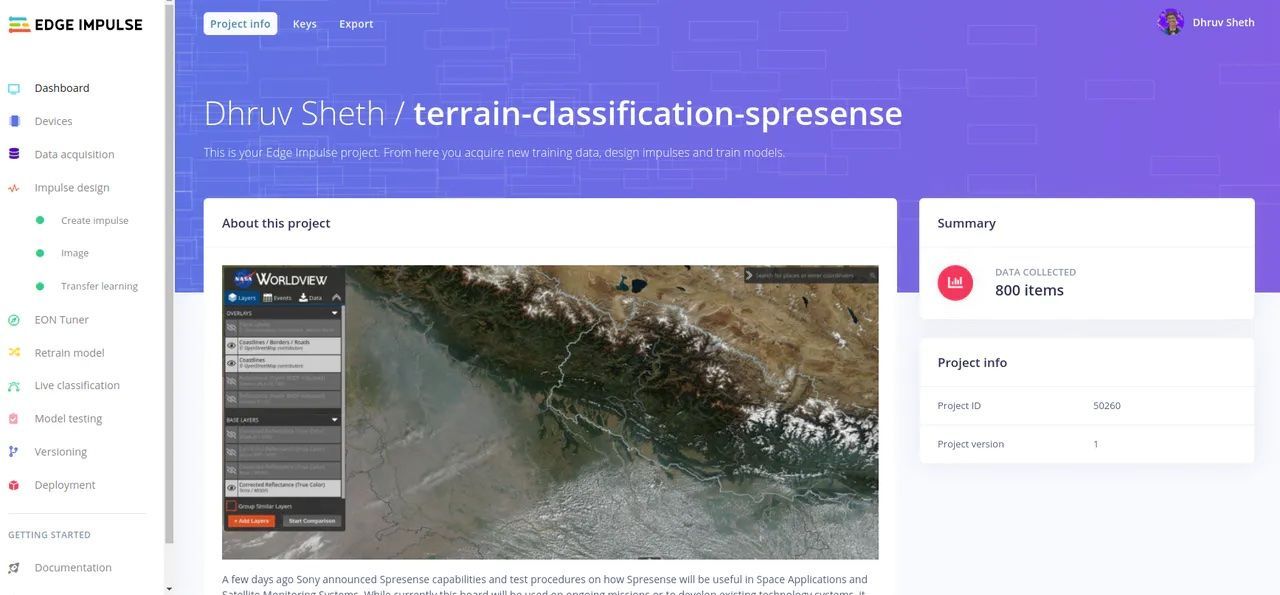
The cloud classification Edge Impulse project has been made public by Sheth, so be sure to check it out for a head start in creating your own version. You can also read his entire write-up for further details.
Want to see Edge Impulse in action? Schedule a demo today.