As the technology landscape continues to evolve, companies are constantly on the lookout for ways to gain a competitive advantage, streamline operations, and drive innovation. Enter machine learning (ML), one of the most impactful technologies that continues to reshape industries, and how companies operate.
What is machine learning (ML)?
At its core, ML is a subset of artificial intelligence (AI) that enables systems to learn and improve from experience without being explicitly programmed to do so. Examples of machine learning include chatbots, predictive text, the shows Netflix suggests to you, and the ability to diagnose medical conditions based on images.
“Machine learning is changing, or will change, every industry, and leaders need to understand the basic principles, the potential, and the limitations.”
—MIT computer science professor Aleksander Madry, director of the MIT Center for Deployable Machine Learning
These capabilities open up a world of possibilities for businesses, from personalizing customer experiences to optimizing supply chains, and detecting fraud in real-time.
How does machine learning work?
Capable of analyzing vast amounts of data, uncovering hidden patterns, and making predictions with remarkable accuracy, ML has emerged as a powerhouse in the technology landscape.
The process typically involves a number of steps:
- Data collection: Gathering relevant, high-quality data for the use case/problem at hand.
- Data preparation: Cleaning, normalizing, and formatting data for analysis.
- Feature extraction: Transforming the data in order to ease training and improve model performance.
- Model selection: Choosing an appropriate algorithm based on the problem type and data characteristics.
- Training: Feeding the prepared data into the chosen algorithm, which then “learns” from the patterns in the data.
- Validation: Testing the model on separate datasets to assess its performance and generalisability.
From tech giants to small start-ups, organizations across the spectrum are harnessing ML to solve complex problems, enhance decision-making, and create new opportunities for growth.
Top Industries and Use Cases Leveraging Machine Learning
The integration of ML into business has experienced a significant uptick in recent years, and the global machine learning (ML) market size is expected to grow from $26.03 billion USD in 2023 to $225.91 billion USD by 2030 .
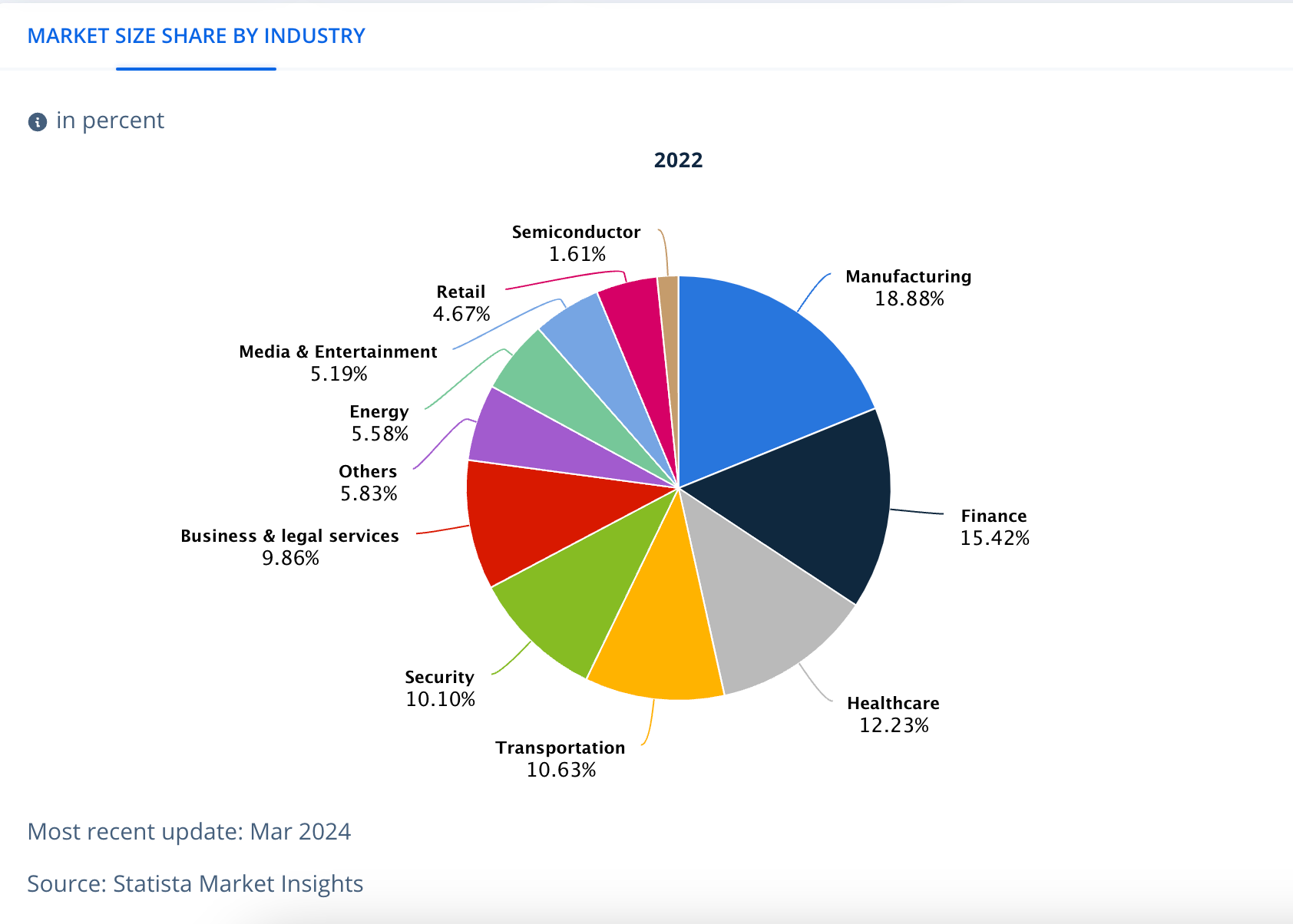
Industries
Manufacturing
Manufacturers are leveraging AI to unlock greater efficiencies within their production environments. They are specifically using ML on local devices to harness real-time insights from sensing technologies.
In a recent Edge Impulse/Manufacturing Dive survey of manufacturing leaders, 100% of those surveyed answered yes to the question of whether their companies were working on initiatives to integrate AI and/or ML into their manufacturing environment.
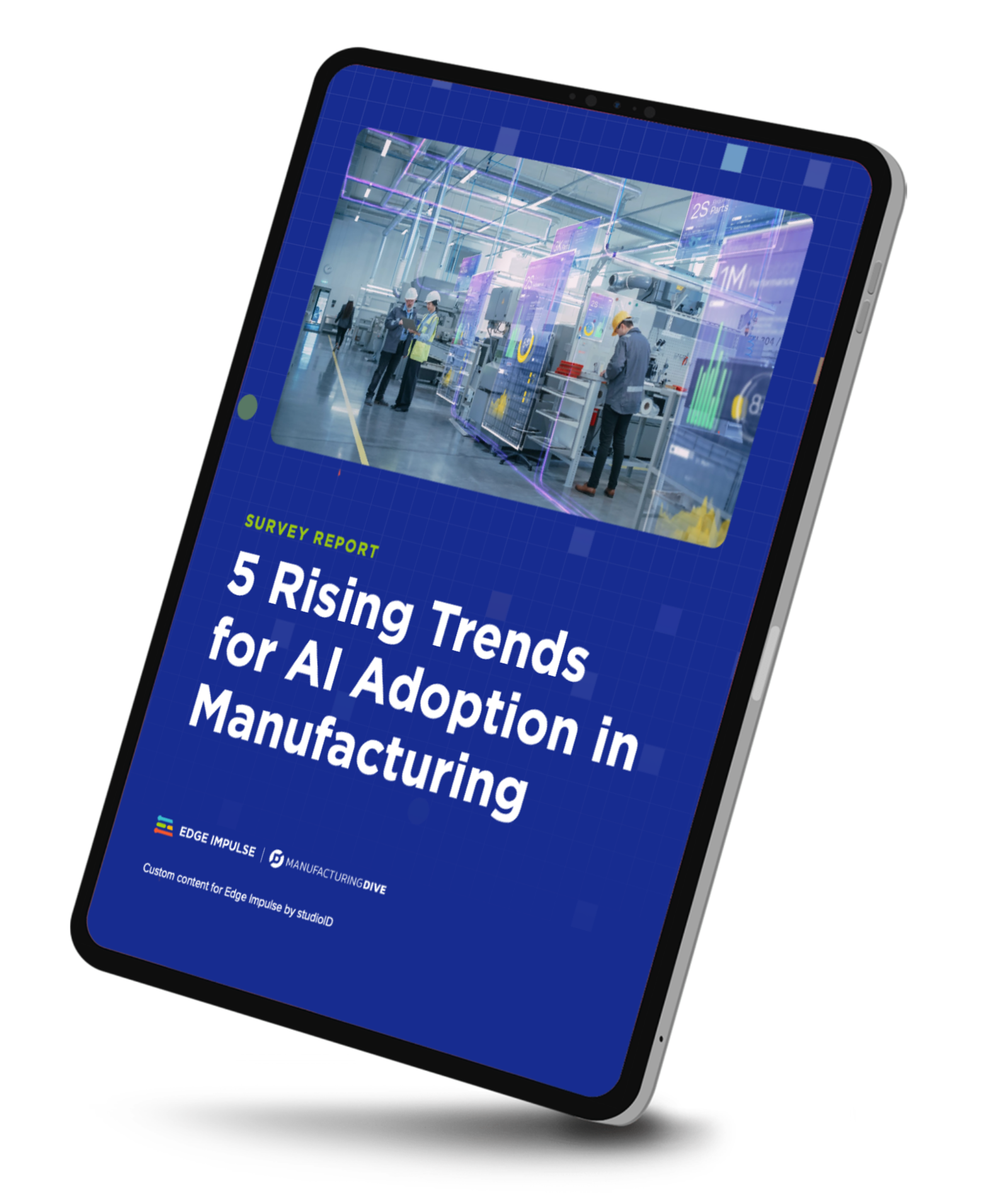
Several AI trends among participants were noted in the survey report, including production optimization, real-time insights from AI to increase profitability, and quality and safety as business drivers.
Healthcare
The healthcare industry has witnessed significant advancements in ML applications, particularly in medical diagnosis, pandemic monitoring, and imaging diagnostics, according to Intuition’s article, Machine learning by the numbers: Its impact on business.
Hospitals and research institutions are leveraging AI algorithms to analyze medical images, detect diseases earlier and predict patient outcomes. For instance, ML models can now identify subtle patterns in mammograms that might escape even experienced radiologists, potentially saving lives through earlier detection of cancer.
The global AI in healthcare market size is predicted to reach around USD 613.81 billion by 2034, growing at a compound annual growth rate (CAGR) of 36.83% from 2024 to 2034.
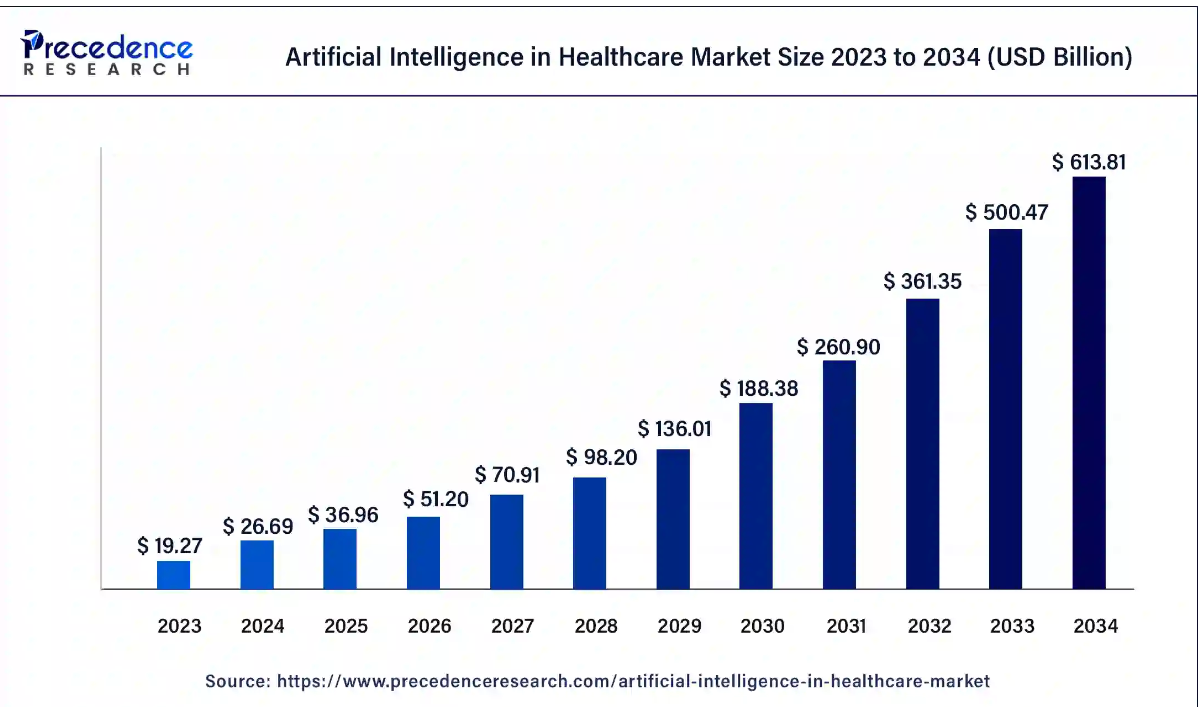
Retail
According to Exploding Topics, 60% of consumers say they’ll become repeat customers after a personalized shopping experience. Retailers leverage ML to personalize customer experiences, optimize inventory management, and predict market trends. These use cases yield many benefits including increased sales, reduced costs, and improved customer satisfaction.
Transportation
ML is driving innovations across the transportation industry; vehicles, infrastructure, and logistics. AI-powered systems enable autonomous vehicles, optimize traffic flow, and enhance predictive maintenance for fleets. For logistics, ML optimizes delivery routes and enhances supply chain visibility.
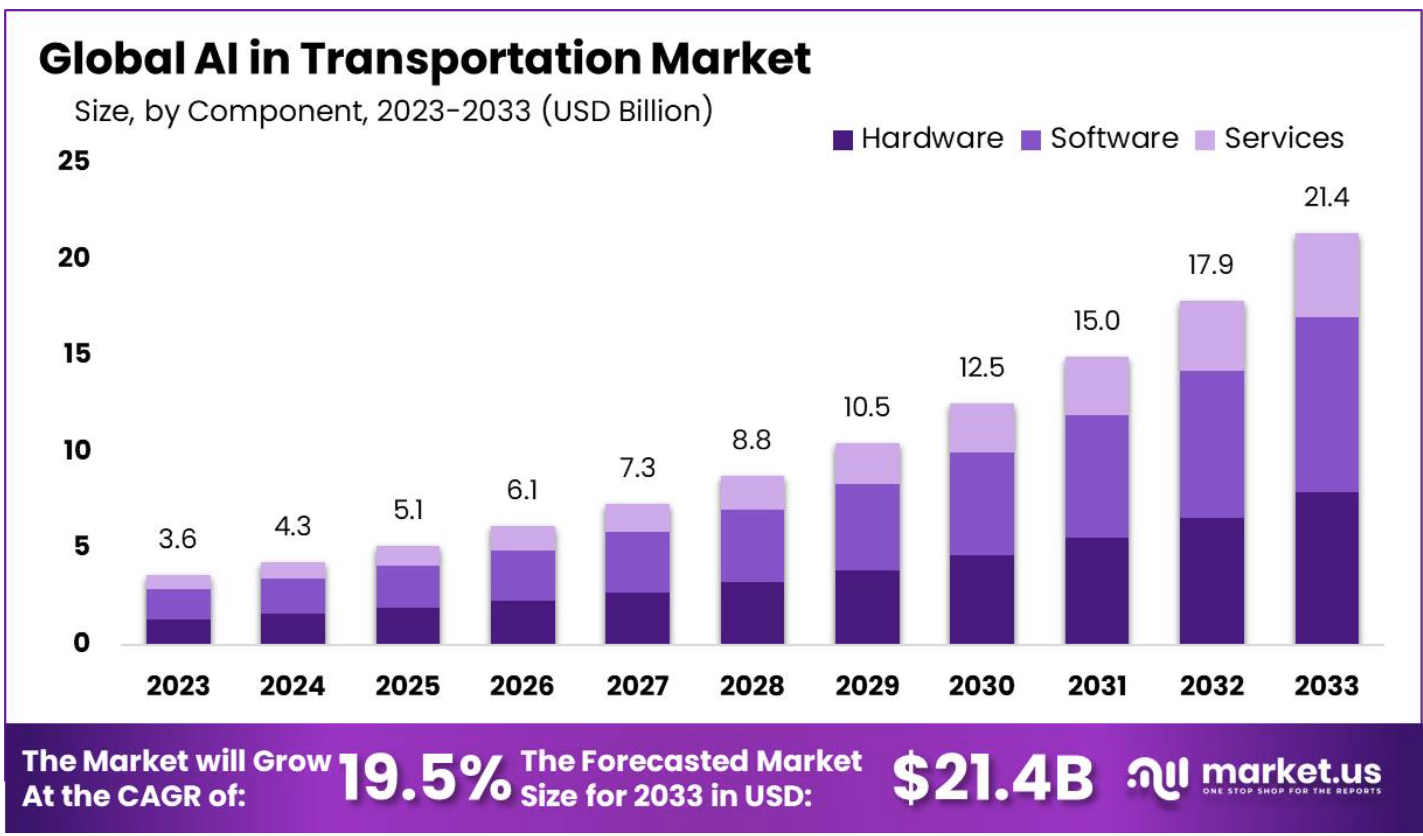
Case studies and use cases
In healthcare, ML aids in disease diagnosis, drug discovery, and personalized treatment plans, while predictive maintenance and quality control take center stage in the manufacturing sector. For those in the energy industry, ML optimizes grid management and predicts equipment failures. These examples are just a tip of the iceberg, as ML applications continue to emerge across virtually every sector.
McKinsey & Company provided some powerful examples of how large organizations are using ML to increase sales and drive efficiency:
- The National Basketball Association (NBA) — teams in the NBA have worked with start-up Second Spectrum, which uses machine learning to digitize teams’ games to create predictive models. The models allow coaches to distinguish between, as CEO Rajiv Maheswaran puts it, “a bad shooter who takes good shots and a good shooter who takes bad shots.”
- More than a dozen European banks have replaced older statistical-modeling approaches with machine learning techniques. In some cases, they’ve experienced 10% increases in sales of new products, 20% savings in capital expenditures, 20% increases in cash collections, and 20% declines in churn.
- Netflix saved $1 billion due to its machine learning algorithm for the combined effect of personalization and content recommendations
- American Express has witnessed a 30% reduction in fraud losses due to deployment of machine learning for predictive analytics
Here are a few ML statistics that demonstrate its importance as a business driver:
- 48% of businesses globally use machine learning
- 20% of C-level executives (across ten countries and 14 different industries) report using machine learning as a core part of their business
- 57% of companies and businesses use machine learning to improve consumer experience
- 49% of the companies use machine learning and AI in marketing and sales
- $3.1B has been raised for machine learning companies with the investments of more than 4400 companies
- Amazon’s average ‘click to ship’ time has been reduced by 225% from 60-75 minutes to 15 minutes
As with any new technology, businesses must assess the ROI before fully committing their resources. Here are a few additional statistics that demonstrate the financial impact of ML:
- 74% of data scientists and C-level executives use ML for performance analysis and reporting.
- 80% of organizations that have used machine learning say the technology has helped their bottom line. Source: Statista
- 42% of companies said the profitability of their ML and AI initiatives exceeded their expectations.
- 14% increase in global GDP by 2030 is forecasted with the advancements of ML and AI.
However, the benefits of machine learning aren’t confined to big businesses. According to the Forbes article 16 Valuable Benefits Machine Learning Can Bring To Small Businesses, small-business owners can leverage the latest ML models and reap multiple benefits including: improved workflows, theft detection, a snapshot of financial health, improved agility and productivity, accelerated time to market and ROI, and protection from phishing attacks.
Challenges and Considerations When Deploying ML Models
Data quality and availability: One of the biggest critical challenges in machine learning is data quality. Models require large amounts of accurate, representative data to perform well.
Data selection: Collecting large amounts of data from various sources can be challenging, especially for large datasets.
Scalability: As model complexity and size of datasets grow, so do the compute resources required for training and inference.
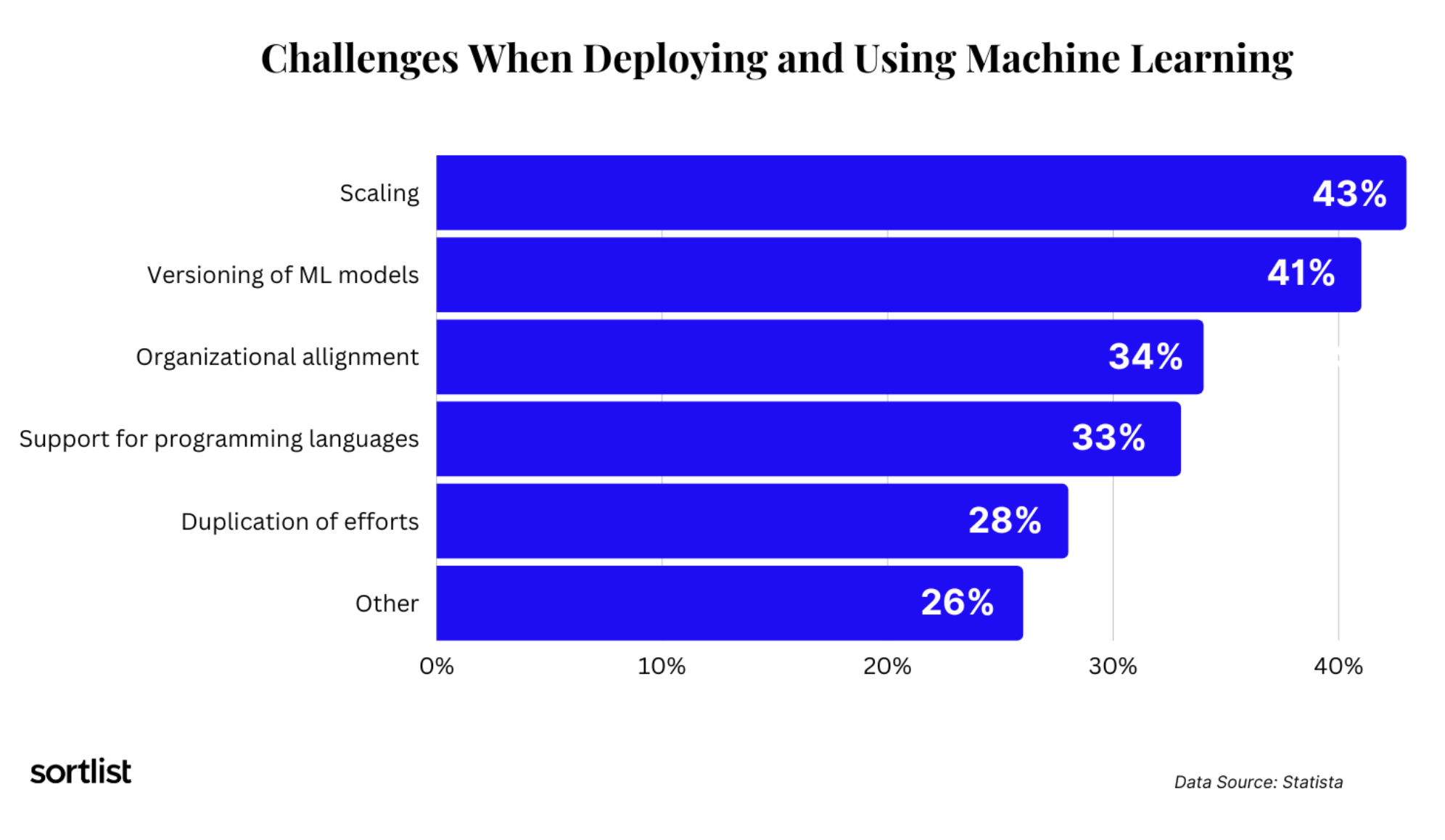
Model optimization: Reducing model size and computational requirements enables sophisticated AI models to run more efficiently on resource-constrained hardware, dramatically expanding the potential applications of edge AI. The benefits of model optimization are multi-fold including:
- Reduced energy consumption, leading to longer battery life
- Decreased memory usage, allowing for more complex functionality on simpler devices
- Lower latency, enabling real-time responsiveness crucial for many edge AI applications
How to Get Started with Machine Learning
Identify use cases or business challenges suitable for ML
Identifying suitable use cases for machine learning involves assessing business challenges through the lens of data availability and problem complexity. Ideal ML applications often involve tasks that are repetitive in nature, data-intensive, and require pattern recognition or prediction.
Start by examining areas where your organization has an abundance of high-quality data and measurable objectives. Ask a lot of questions: Are there processes that rely on manual analysis? Do you have problems where slight improvements in accuracy or efficiency could yield significant business value? Remember to evaluate the potential impact against the resources required for implementation.
Build a data strategy
A comprehensive data strategy aligns data management and utilization with overall business objectives. It begins with assessing current data assets, infrastructure and capabilities. Not enough data? Synthetic data is available on the Edge Impulse platform, enabling a new and efficient way to work with LLM-generated images, audio, and voice to enhance your edge AI models.
Next, define clear goals for data usage, such as improving decision-making, enhancing customer experiences, or driving innovations.
Establish data governance policies to ensure data quality, security, and compliance with regulations. And of course, invest in the right technologies and tools for data collection, storage, processing, and analysis.
Choose the right tools and platforms
Choosing the right tool is crucial for successful implementation. Begin by evaluating your specific needs and the type of ML problem you're addressing, your team’s technical expertise, and scalability requirements.
There are a number of platforms you can consider including Edge Impulse, AWS Sagemaker, Azure Machine Learning, and TensorFlow. Look for platforms that integrate well with your existing data infrastructure. Also factor in community support and documentation available for each tool.
By capitalizing on the power of data and advanced algorithms, you can gain deep insights, automate complex processes, and make better informed decisions. The potential applications of ML span across multiple industries, but its true power might lie in its ability to drive business value when aligned with clear objectives, and integrated thoughtfully into existing processes.
The key to success is starting small, learning continually, and scaling strategically. By doing so, businesses can unlock new opportunities, enhance customer experiences, and gain a sustainable competitive edge in the era of AI.