Only for specific jobs
Just a few decades ago, headsets were meant for use only with specific job functions – primarily B2B. They were used as simply extensions of communication devices, reserved for astronauts, mission control engineers, air traffic controllers, call center agents, fire fighters, etc. who all had mission critical communication to convey while their hands had to deal with something more important than holding a communication device. In the B2C consumers space you rarely saw anyone wearing headsets in public. The only devices you saw attached to one’s ears were hearing aids.
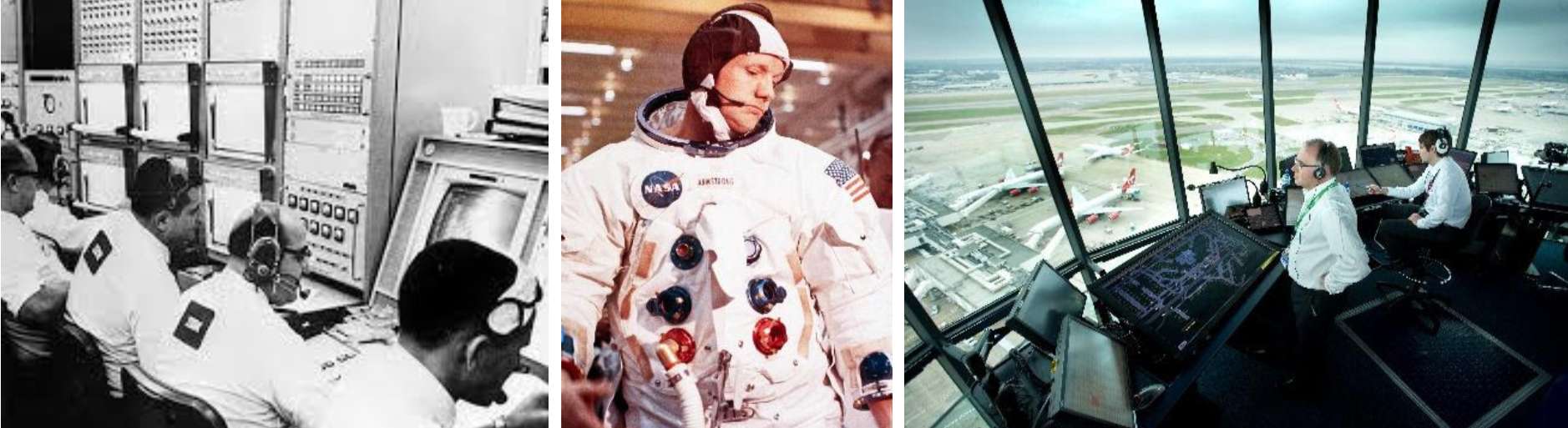
Tale of two cities: Telephony and music
Most headsets were used for communication purposes, which also referred to as ‘Telephony’ mode. As with most communications, this requires bi-directional audio. Except for serious audiophiles and audio professionals, headsets were not used for music consumption. Any type of half-duplex audio consumption was referred to as ‘Music’ mode.
Deskphones and speakerphones
Within the enterprise, a deskphone was the primary communication device for a long time. Speakerphones were becoming a common staple in meeting rooms, facilitating active collaboration amongst geographically distributed team members. So, there were ‘handsets’ but no ‘headsets’ quite yet.
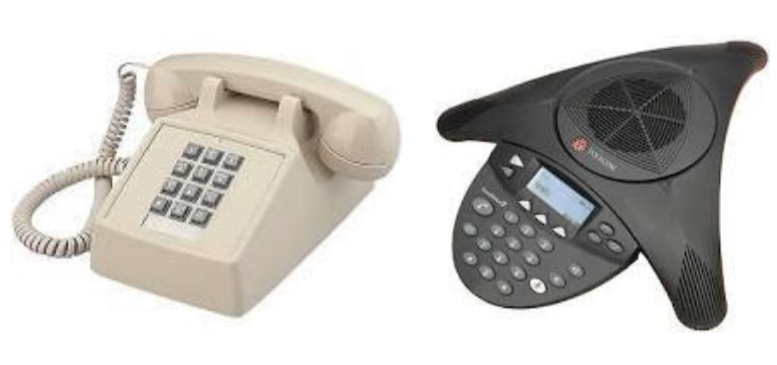
Mobile revolution: Communication and consumption
As the Internet and the browser were taking shape in the early ’90s, deskphones were getting untethered in the form of big and bulky cellular phones. At around the same time, a Body Area Network (BAN) wireless technology called Bluetooth was invented. Its original purpose was simply to replace the cords used for connecting a keyboard and mouse to the personal computer.
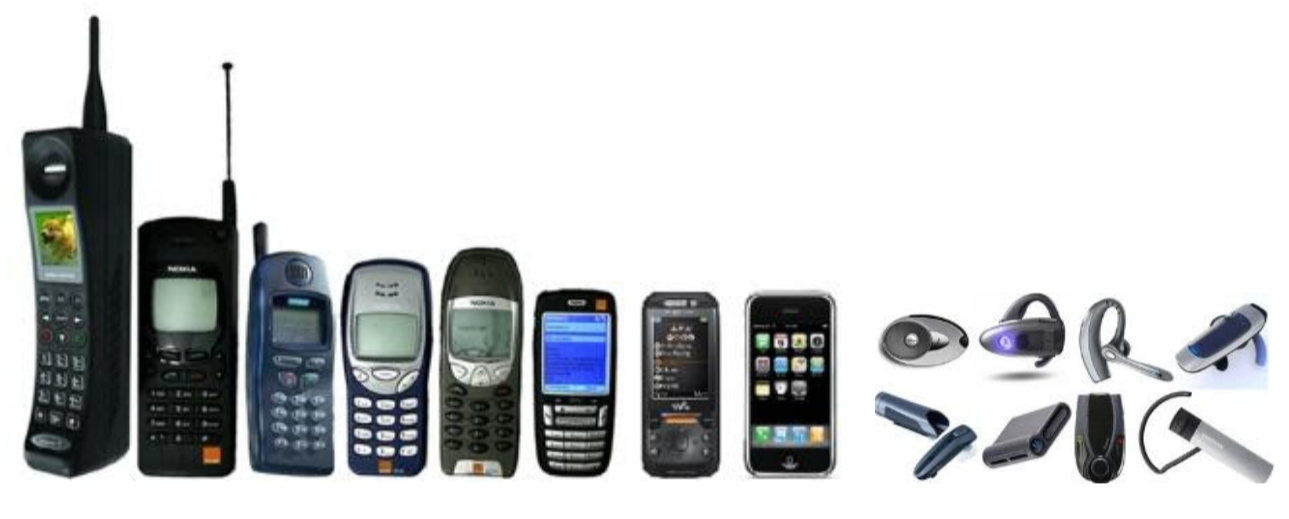
As cellular phones were slimming down and becoming more mainstream, scientists figured out how to use Bluetooth radio for short-range full-duplex audio communications as well. Fueled by rapid cell-phone proliferation, along with the need for convenient hands-free communication by enterprise executives and professionals (for whom hands-free communication while being mobile was important), monaural Bluetooth headsets started becoming a loyal companion to cell phones.
While headsets were used with various telephony devices for communications, portable analog music (Sony Walkman, anybody?) started giving way to portable digital music. Cue the iPod era. The portable music players primarily used simple wired speakers on a rope. These early ‘earbuds’ didn’t even have a microphone in them because they were meant solely for audio consumption – not for audio capture.
The app economy, softphones and SaaS
Mobile revolution transformed simple communication devices into information exchange devices and then more recently, into mini super computers that have applications to take care of functions served by numerous individual devices like a telephony device, camera, calculator, music player, etc. As narrowband networks gave way to broadband networks for both the wired and wireless worlds, ‘communication’ and ‘media consumption’ began to transform in a significant way as well.
Communication: Deskphones or ‘hard’-phones started being replaced by VoIP-based soft-phones. A new market segment called Unified Communications (UC) was born because of this hard- to soft-phone transition. UC has been a key growth driver for the enterprise headset market for the last several years, and it continues to show healthy growth. Enterprises could not part ways with circuit-switched telephony devices completely, but they started adopting packet-switched telephony services called soft-phones. So, UC communication device companies are effectively helping enterprises by being the bridge from ‘old’ to ‘new’ technology. UC has recently evolved into UC&C – where the second ‘C’ represents ‘Collaboration.’ Collaboration using audio and video (like Zoom or Teams calls) got a real shot in the arm because of the COVID-19-induced remote work scenario that has been playing out globally for the last year and a half.
Media consumption: ‘Static’ storage media (audio cassettes, VHS tapes, CDs, DVDs) and their corresponding media players, including portable digital music devices like iPods, were replaced by ‘streaming’ services in a swift fashion.
Why did this transformation matter to the headset world?
Communication & collaboration by the enterprise users as well as media consumption by consumers collided head-on. Because of this, monaural headsets have almost become irrelevant. Nearly all headsets today are binaural or stereo, and have microphone(s) in them.
This is because the same device needs to serve the purposes of both: consuming half-duplex audio when listening to music, podcasts, or watching movies or webinars, and enabling full-duplex audio for a telephone conversation, a conference call, or video conference.
Fewer form factors… more smarts
From: Very few companies building manifold headset form factors that catered to the needs of every diverse persona out there.
To: Quite a few companies (obviously, a handful of them a great deal more successful than the others) driving the headset space to effectively just two form factors:
- Tiny True Wireless Stereo (TWS) earbuds and
- Big binaural occluding cans!
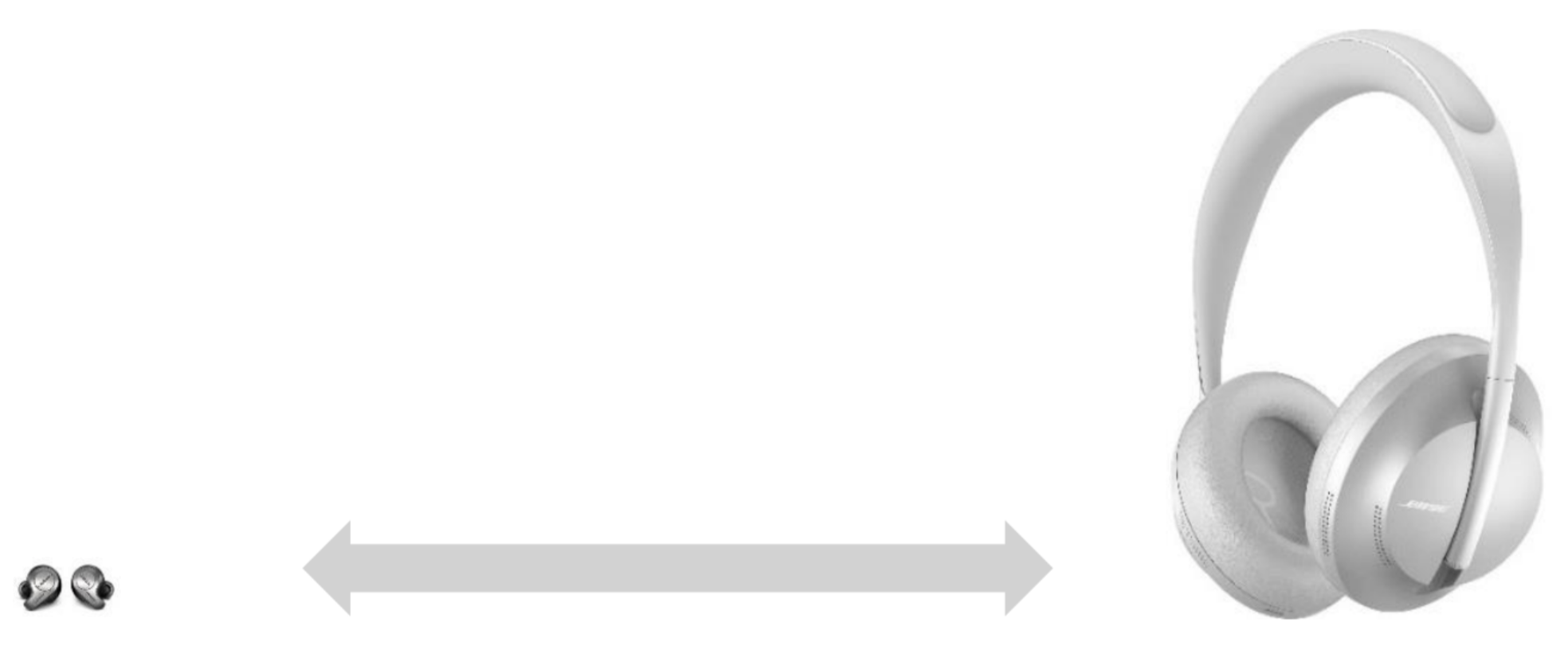
Less hardware… more software
Such a trend has been in place for quite some time impacting several industries. Headsets are no exception. Ever so sophisticated semiconductor components and proliferation of miniaturized Microelectromechanical Systems, or MEMS in short, components have taken the place of numerous bulkier hardware components.
What do modern headsets primarily do with regards to audio?
- Render received audio in the wearer’s ear
- Capture spoken audio from the wearer’s mouth
- Calculate anti-noise and render it in the wearer’s ear (in noise-cancelling headsets)
Sounds straightforward, right? It is not as simple as it sounds – at least for enterprise-grade professional headsets. Audio is processed in the digital domain in all modern headsets using sophisticated digital signal processing techniques. DSP algorithms running on the DSP cores of the processors are the most compute-intensive aspects of these devices. Capture/transmit/record audio DSP is relatively more complicated than the render/receive/llayback audio DSP. Depending on the acoustic design (headset boom, number of microphones, speaker/microphone placement), audio performance requirements, and other audio feature requirements, the DSP workload varies.
Intelligence right at the edge!
Headsets are true edge devices. Most headset designs have severe constraints around several factors: cost, size, weight, power, MIPS, memory, etc.
Headsets are right at the horse’s mouth (pun intended) of massive trends and modern use cases like:
- Wake word detection for virtual private assistants (VPAs)
- Keyword detection for device control and various other data/analytics purposes
- Modern user interface (UI) techniques like voice-as-UI, touch-as-UI, and gestures-as-UI
- Transmit noise cancellation/suppression (TxNC or TxNS)
- Adaptive ambient noise cancellation (ANC) mode selection
- Real-time transcription assistance
- Ambient noise identification
- Speech synthesis, speaker identification, speaker authentication, etc.
Most importantly, note that there is immense end customer value for all these capabilities.
Until recently, even if one wanted to, very little could be done to support most of these advanced capabilities right in the headset. Just the features and functionalities that were addressable within the computational limits of the on-board DSP cores using traditional DSP techniques were all that could be supported.
Enter edge compute, AutoML, tinyML, and MLOps revolutions…
Several DSP-only workloads of the past are rapidly transitioning to an efficient hybrid model of DSP+ML workloads. Quite a few ML only capabilities that were not even possible using traditional DSP techniques are becoming possible right now as well. All of this is happening within the same constraints that existed before.
Silicon as well as software innovations are behind such possibilities. Silicon innovations are relatively slow to be adopted into device architectures at the moment, but they will be over time. Software innovations extract more value out of existing silicon architectures while helping converge on more efficient hardware architecture designs for next-generation products.
Thanks to embedded machine learning, tasks and features that were close to impossible are becoming a reality now. Production-grade inference models with tiny program and data memory footprints in addition to impressive performance are possible today because of major advancements in AutoML and tinyML techniques. Building these models does not require massive amounts of data either. The ML-framework and automated yet flexible process offered by platforms like those from Edge Impulse make the ML model creation process simple and efficient compared to traditional methods of building such models.
Microphones and sensors galore
All headsets feature at least one microphone, and many feature multiple, sometimes up to 16 of them! The field of ML for audio is vast, and it is continuing to expand further. Many of the ML inferencing that was possible only at the cloud backends or sophisticated compute-rich endpoints are now fully possible in most of the resource-constrained embedded IoT silicon.
Microphones themselves are sensors, but many other sensors like accelerometers, capacitive touch, passive infrared (PIR), ultrasonic, radar, and ultra-wideband (UWB) are making their way into headsets to meet and exceed customer expectations. Spatial audio, aka 3D audio, is one such application that utilizes several sensors to give the end-user an immersive audio experience. Sensor fusion is the concept of utilizing data from multiple sensors concurrently to arrive at intelligent decisions. Sensor fusion implementations that use modern ML techniques have been shown to have impressive performance metrics compared to traditional non-ML methods.
Transmit noise suppression (TxNS) has always been the holy grail of all premium enterprise headsets. It is an important aspect of enterprise collaboration. A magical combination of physical acoustic design – which is more art than science – combined with optimally tuned complex audio DSP algorithms implemented under severe MIPS, memory, latency, and other constraints. In recent years, some groundbreaking work has been done in utilizing recursive neural network (RNN) techniques to improve TxNS performance to levels that were never seen before. Because of their complexity and high-compute footprint, these techniques have been incorporated into devices that have mobile phone platform-like compute capabilities. The challenge of bringing such solutions to the resource-constrained embedded systems, such as enterprise headsets, while staying within the constraints laid out earlier, remains unsolved to a major extent. Advancements in embedded silicon technology, combined with tinyML/AutoML software innovations listed above, is helping address this and several other ML challenges.

Conclusion
Modern use cases that enable the hearables to become ‘smart’ are compelling. Cloud-based frameworks and tools necessary to build, iterate, optimize, and maintain high performance small footprint ML models to address these applications are readily available from entities like Edge Impulse. Any hearable entity that doesn’t take full advantage of this staggering advancement in technology will be at a competitive disadvantage.