Edge Impulse has partnerships with a great range of silicon vendors and chipmakers, providing native software support for devices from these companies. One notable Edge Impulse partner is BrainChip, the Australia-based company that has created a proprietary neuromorphic processor, called AkidaTM, using spiking neural networks that replicates human brain activity. The nature of neuromorphic processing makes it especially well-suited for artificial intelligence applications, which makes BrainChip and Edge Impulse a great combination.
With the close connection between both teams, Edge Impulse’s Solutions Engineering Manager Joshua Buck sat down with BrainChip CMO Nandan Nayampally to talk through the underlying technology that they’re creating, the areas that the company is focusing on, some of the latest developments, and more.
Josh Buck: Hello Nandan. I'd like to hear about the newly released Edge AI Box that features the Akida AKD1000 neuromorphic processor. But first, what is BrainChip’s chief differentiator in the market? Why do you guys exist and what are you hoping to accomplish?
Nandan Nayampally: Josh, thank you for having me. Part of the reason we are doing this is to accelerate what BrainChip believes is our key differentiator, which is extremely low-power processing for more complex neural networks out at the edge without the need to connect the cloud.
BrainChip, as the name suggests, is inspired by the brain, which is one of the most efficient computational, inferential, and predictive engines known. The neuromorphic approach relies on the behaviors of neurons, which only fire when needed, and hence are more efficient with computation itself, and with how much is needed. That's where BrainChip derives its inspiration. We call it neuromorphic processing, but really event-based processing. And the minor difference here is, traditionally neuromorphic aims to be analog, which is great, but at the same time is much more difficult to achieve and not as portable. BrainChip has gone the approach of building an event-based, purely digital version of it, which can actually be sold as IP, or licensed as IP to go into lots of different edge solutions.
The other advantage that you have with BrainChip: While neuromorphic computing and what is traditionally called spiking neural nets, which truly mimic brain function, are cool, they are not yet production-ready and are not as prevalent in the marketplace. So, what BrainChip has done with its event-based processor is to make it much easier to take today's models — convolutional transformers, etc. — and make them run on hardware that is much more event-based. So key advantages are that it can be extremely power efficient on complex models, you can do them in battery-operated or very low thermal environments, completely disconnected from the cloud if needed. And that really changes how you can bring more compelling, intelligent applications to the edge.
Josh Buck: What are the key challenges that we're trying to solve for the customers in this space? You've already mentioned a few, like vision applications, but in particular with the Edge Box? And how does the partnership with Edge Impulse supporting this Edge Box help?
Nandan Nayampally: Edge Impulse has taken on the mantle of being a development platform for AI at the edge, which, when it started a few years ago, I thought was a very big undertaking, because it's a very fragmented space. And what you guys have done in terms of building a developer community with the projects that people are creating, is an amazing validation of the fact that there is real hunger to do things at the edge. And the reason, of course, is the fact that the demands on the cloud are overwhelming, and if anything, generative AI has taken an order of magnitude more complex and the cloud can't keep up. So you do need to pull a lot of that to the edge.
What you guys have done in terms of building a developer community with the projects that people are creating, is an amazing validation of the fact that there is real hunger to do things at the edge.
Now “edge” means a lot of things to a lot of people as you know. BrainChip’s technology goes from what we call far, far edge or sensor-edge, where it's right in sub-watt or sub-milliwatt-types of applications, very close to sensor, IP integrated, all the way up to the network edge where it's almost a server-type application, but not quite. And so, the Edge Box from VVDN sits closer to the right-hand side, if you will, closer to the network edge. But the goal is to actually have a very compact, portable, scalable, cost-effective box that can enable lots of interesting applications. For example, if you have multiple video streams coming in retail, where you're managing the store, that can be computed on the box itself. The box will have connectivity with Ethernet with Wi-Fi, etc., but the main part is it's going to do the heavy lifting of the compute in real time. One of the key aspects of edge is you want these things to be real-time, independent of how good your connection to cloud is. So the real critical part needs to be done close to where it is.
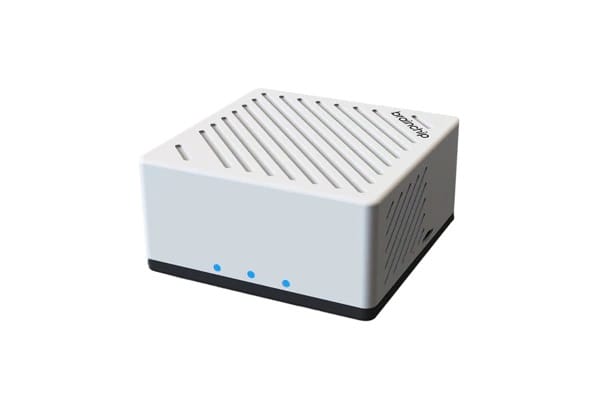
Josh Buck: Absolutely, I see that a lot too. In my role at Edge Impulse, I work directly with customers both on the far edge and, like you said, server edge. In both scenarios, and even the ones in between, it's a lot about making sure that we can prove that the data is valuable, and that you don't have to ship it off to the cloud. You can adopt processing on site and get real-time insights directly there, whether or not you have cloud connectivity. My experience with using the AKD1000, which is inside this Edge Box, has been that it's been able to do some pretty high tech models. We can do object detection, we can do image classification, and also some of the sensor-based applications.
What I've been really excited to explore with this device is how we can enable customers to show that the data they have today, even if it's in the cloud or not, is worth something, and they can run it on all these devices very quickly and efficiently. With some of the integrations that we have already with BrainChip, and with stuff that's coming out in the future, they'll be able to test out their models, test out their data, and then get performance insights. And then when these devices are available, they'll be able to actually run them and then validate that they have this data here that works well. And they don't have to extricate it to the cloud just to get the inferencing that they need.
Nandan Nayampally: That's well put, Josh. I think the real benefit that we see is that the idea of completely integrated AI into very, very small sensors will take time. It is probably where it should be just because of the fact that you can do it in real time and minimize the amount of communication needed to minimize the amount of context-free computation. At every stage you go further up the network, you lose some context, and you have to put more brute force in it. So, the true distributed hybrid approach is where it will gravitate to, but you will find those points. What the Edge Box does is give you that point that is on the edge side of the network edge. It gives you the ability to, at the same time, have computation.
The Edge Box has an Arm-based processing unit with GPU from NXP, an iMX 8-based one. So, you have computation, user experience, as well as some meaningful intelligence being built into your solution. For example, in the retail space, can you actually understand if your stacking of shelves is appropriate? Can you understand customer behaviors? Can you understand potential threats? Those are all things that people would like to see. But the cost of getting a cloud service for that is pretty high. And the response may not be timely enough. You see the same thing for healthcare. Can you actually manage health care networks, especially remotely? That's another area where the Edge Box comes in handy. We see that for smart city, we see that for automotive transportation, etc.
I think this enables a lot of different types of smaller businesses to bring this to market in small-volume configurations and make it still cost effective versus trying a much heavier cloud or network edge solution.
In the retail space, can you actually understand if your stacking of shelves is appropriate? Can you understand customer behaviors? Can you understand potential threats? Those are all things that people would like to see.
Josh Buck: Tell me more about the specifics on the Edge Box. You mentioned it’s an iMX device, I assume it has Linux on it, it will have the AKD1000. Can you share some of the production ready-specs that enable it to go out in the field or is that to be released?
Nandan Nayampally: We released some of those specs this past February, with the launch of the pre-sale. These are at a datasheet level, if you will. I think in terms of the actual performance metrics on types of models, we would like our partner VVDN to present it, because they're the ones delivering the QoS for it, but we'd expect to consistently be delivering more and more information as we go along. But there's a lot of excitement around it, and I think most users will look at this or developers will look at it as a great way to do POCs and then go into small-volume production before they get to the next step of higher volume solutions.
Josh Buck: Edge Impulse wants to be right there in that journey with you. The type of things that we provide already for the Akida platform show off some of the models that you have today on BrainChip’s website, and even some of the Edge Impulse applications on there. There's been a number of developments with FOMO — Edge Impulse’s model for object detection — that works very well in the BrainChip AKD1000. And with that enabled on the Edge Box now, we're just one more step to bringing this to production so that people can literally drop it into place and get started very fast, and then go to that kind of small-volume production, and have that data set ready that they may already have and get it onto the field and into production quickly. I’m really excited about that.
Nandan Nayampally: Edge Impulse, I feel, is an important cog in the wheel. We're happy to be the first IP partner that Edge Impulse has worked with, and we've worked very closely on that. And then having this for the Box gives the developers a spectrum where they can use the Edge Box. They can use our development boards, and beyond the boards, when our partners come to market with integrated Akida solutions, they have a common development platform for all three. And that actually gives a developer continuity in a usage familiarity, which is important because as everyone knows, it's a pretty complex environment.
When we started, we assumed that everyone would be a super user using TensorFlow or PyTorch. That's only a small section of the developer community. Our MetaTF software supports that. For the people that are doing low-code/no-code, like yourselves, or enabling low-code yourself, I think Edge Impulse has been a great platform for us to integrate our compilation technology tools in, so that they can build on a much more stable environment and build out less intense development cycles, but there's a ready set of widgets for them to choose from and optimize thereafter. And then, of course, we're also working with application vendors such as NVISO and Ai Labs, which are solving [vehicle] in-cabin or factory maintenance or such problems for direct users that can solve direct applications.
Edge Impulse has been a great platform for us to integrate our compilation technology tools in, so that they can build on a much more stable environment and build out less intense development cycles, [with] a ready set of widgets to choose from
Josh Buck: It's good to hear that you have a whole ecosystem and a zero to 100 solution. Not only can you start off and go down to the IP level, and integrate it into your chips and sensors that you need to, but then you have, development boxes, like the enablement platforms you have today, the new Edge Box for production, then you have software support from MetaTF, which is what you provide for the coding environment. And then people can build on top of that, like we did at Edge Impulse, for production solutions and things like that. And then you have partners as well, to go target real specific applications, maybe as a system integrator, if you will, to bring a whole solution together if the customer themselves are not able to put all the pieces.
Nandan Nayampally: Exactly. And in fact, if you think about VVDN, the Edge Box, it is us working with the ecosystem, yourselves, VVDN, you could put them somewhere between an ODM or original device manufacturer or a system integrator. This is not the business that we're in. BrainChip is not an Edge Box builder; BrainChip is enabling a lot of the Edge Box vendors to come up with meaningful, clear solutions that they can then take to their channel and proliferate efficient edge AI processing.
Josh Buck: So, what's next for BrainChip? When do you expect the next announcement for Edge Box, upcoming new releases that you're able to talk about, any conferences that we may be at?
Nandan Nayampally: I think one of the key things to understand is that the Edge Box that we're talking about today is still based on our first generation technology. Currently, with the AKD1000, could it be extended to the AKD1500, are those really viable solutions?
What we announced and delivered at the end of 2023 is the second generation, which is a lot more capable. It has some of the things we learned from the market, saying “hey, we need 8-bit processing for sure,” even though neuromorphic can be very efficient and capable with 4 bits or even 2 bits.
We've added in the ability to do more complex models like ResNet 50, but really, we had two big new additions. We can do temporal event-based neural nets. That's a new step for traditionally what I call state-based structured models. And what that does is, for traditional recurrent or even transformer-type models where attention is needed, you can actually build models that are much more compact, much more efficient, but equally as accurate and sometimes, as in this case, more accurate. If you could reduce some of the video object detection by 50x in terms of the model size, 50x in terms of the number of operations needed, while maintaining accuracy, that's a boon for edge computing.
We have also added vision transformer encoder in hardware, which again accelerates a lot of this capability for vision object detection. We see a second generation being very compelling for vision and video, obviously, because that's where a large part of the market is for predictive sequence prediction, such as for predictive maintenance, or a lot of the time series data or sequential data, multi-dimensional data for multimodal data, we see that solution being very compelling. Can it start helping with things like gen AI, can it starts helping with multimodal AI? Absolutely. Obviously, it’s a huge space with potentially very complex models, but we're looking at what parts of it can we accelerate for the edge, speech to text, text to speech, some level of automatic speech, speech recognition. All of those things are possible with the generation two. And we'll be talking more about it as more details come out.
Josh Buck: So new technologies come out, BrainChip, and Edge Impulse definitely work together to exhibit those on Edge Impulse and make sure that customers have access to the tools they need to quickly get to production, like with a VVDN box and other devices that may come out.
Nandan Nayampally: One of the things that we didn't touch on, which is kind of a unique capability, I believe, that is now coming into its own is the ability to learn on device. People confuse it with training, this is not training, it is about taking characteristics and features extracted from training, which are now in the inference model, and being able to customize it. So, if you have a face detection capability that can say, “hey, this is Josh's face,” or none in space, that can be customized on device. And what I saw at CES this year was the ability of doing that customization, that personalization is really important as AI starts coming closer and closer to the edge. People are worried about privacy, people are worried about security, this is going to be important.
Josh Buck: Because when you're customizing the model on device, that doesn't have to go back to the cloud. That privacy and security aspect is what stays and what data happens to it, you can get that personalization, and do this edge learning on device. Enter a mode, give it a few samples, and there, it's now personalized for that particular face or voice or sensor application that you never want to have the potential to even get outside of that box. That's great.
Nandan Nayampally: Yeah. If you were at CES you would have noticed that AI was pretty much everywhere, from intelligent toilets to robots doing anything you want. Pretty much edge AI was there. Now, that doesn't mean that everyone will have an accelerator in it. But the fact is models, requirements, and the need for intelligence at the edge is increasing. And for Edge Impulse’s perspective, it's perfect because you're independent of hardware. You are developer models that can scale to partner A's platform, to partner B's platform, which is an excellent thing to see for you guys, how you actually take it to market. We are excited because that says that there will be more demand for intelligent compute, very efficient compute, that starts pulling these things in.
Josh Buck: Any conferences coming up? We're going to Hardware Pioneers and Embedded World in April.
Nandan Nayampally: I believe we're jointly going to the Hardware Pioneers conference. We obviously will be attending the TinyML. We will be at Embedded World, as we should. Naturally the Edge Vision Alliance summits in May, and there's more, but I think for the next two to three months, we have a pretty full slate of events where Edge Impulse and BrainChip can show customers what's done.
Josh Buck: Thank you Nandan, I appreciate your time.
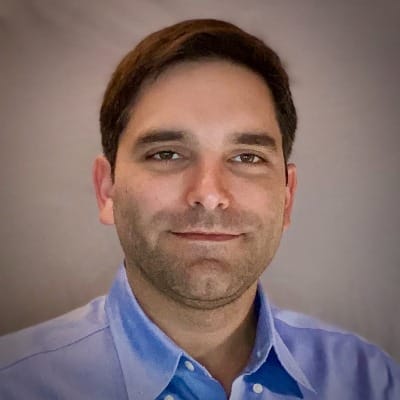
Joshua Buck is an embedded developer with experience in test and measurement, HiL/SiL for automotive, and industrial IoT applications. He has previously worked at National Instruments in applications engineering, analog ASIC V&V, and project management. After National Instruments, he moved into test engineering and embedded development at American Innovations. He is currently a Solutions Engineering Manager at Edge Impulse specializing in AI/ML applications for edge and embedded devices.
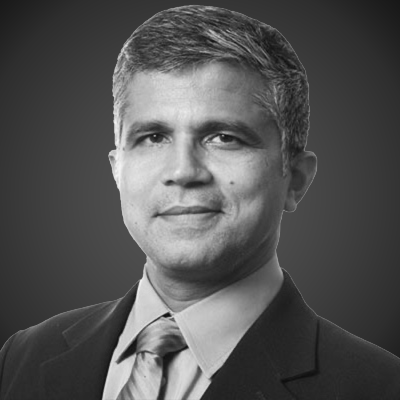
Nandan Nayampally is an entrepreneurial executive with over 25 years of success in building or growing disruptive businesses with industry-wide impact. Nandan was most recently at Amazon leading the delivery of Alexa AI tools for Echo, FireTV and other consumer devices. Prior to that he spent more than 15 years at Arm Inc. including roles as GM developing Arm’s CPU and broader IP portfolio into an industry leader that is built into over 100B chips. He started his career at AMD on their very successful Athlon processor program. He also helped grow product lines in startup businesses such as Silicon Metrics and Denali Software which had successful acquisitions down the road.
Nandan has a B.Tech in Computer Science and Engg from the Indian Institute of Technology, Mumbai and an MA in Computer Science from the University of Texas at Austin.