Machine learning and cutting-edge silicon devices are transforming preventive healthcare
Machine learning and artificial intelligence (AI)-based methods have made a qualitative leap in recent years as a result of new computing software hardware infrastructure and the development of large zettabyte-era databases. These technologies, which serve as the foundation for consumer devices such as smart wearables, smartwatches, smart rings, and smartphones, have resulted in incredible progress on our daily lives, and are poised to transform other areas of healthcare, including clinical research. Here are the ways that Edge Impulse and its trusted suite of ML tools integrates into this segment, and how you can leverage them to help accelerate and amplify your health solution deployments.
Digital biomarkers are the next goldmine for preventative health and wellness companies
Digital biomarkers are quantifiable, measurable indicators that can be collected using devices. They can allow preventive insights to indicate whether a person is healthy or not by measuring blood glucose level along with other biometric parameters like heart rate and pressure.
Increasingly diverse digital biomarkers datasets coming from wearables will enable a whole new generation of preventive healthcare in front of us. Therefore, there has been increased interest in clinical research towards applications that use machine learning models to predictively understand one’s state.
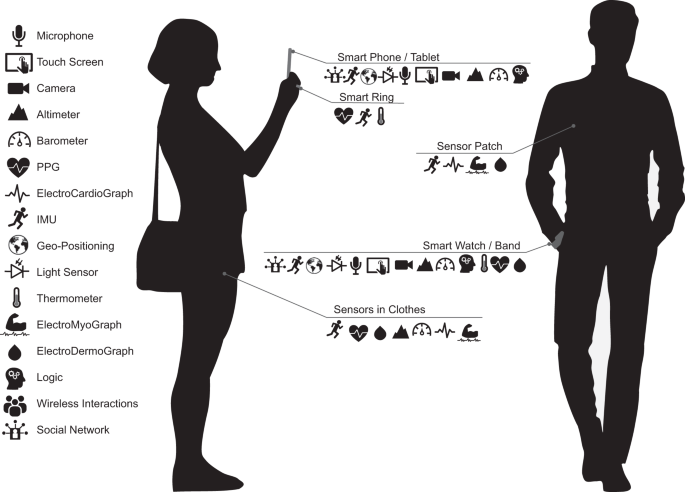
Managing diverse datasets for model development
Technical teams face difficulties in managing and organizing digital biomarkers for model training, which is a crucial process of scaling up the data to train models. These problems can be solved by using a platform that has all these capabilities:
- Centralized cloud storage for AI training that is compatible with different data silos and file types
- Data labeling tools compatible with audio, visual, and sensor-based digital biomarkers
- An integrated algorithm development toolset with no need to go back-and forth between different applications
- Ability to build embedded models optimized for any hardware device
Edge Impulse’s scalable approach to health ML engineering
Edge Impulse already works with companies in the health device space like Nowatch, Oura, SlateSafety, and Knowlabs on their full data engineering and machine learning algorithm development process.
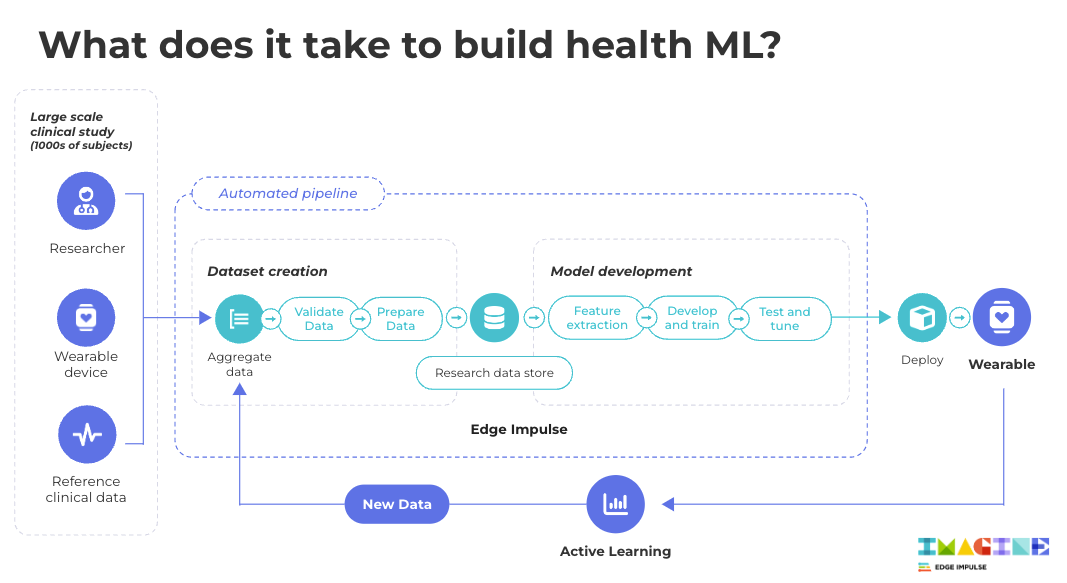
We offer a platform approach for this process to ensure that data is labeled properly, and that algorithms are built against data being collected from gold standard tests to ensure accuracy. Furthermore, algorithms can be tested and improved over time, and even personalized. This is especially critical for health-related algorithms to detect conditions such as fatigue or stress levels where variations between physiology may exist.
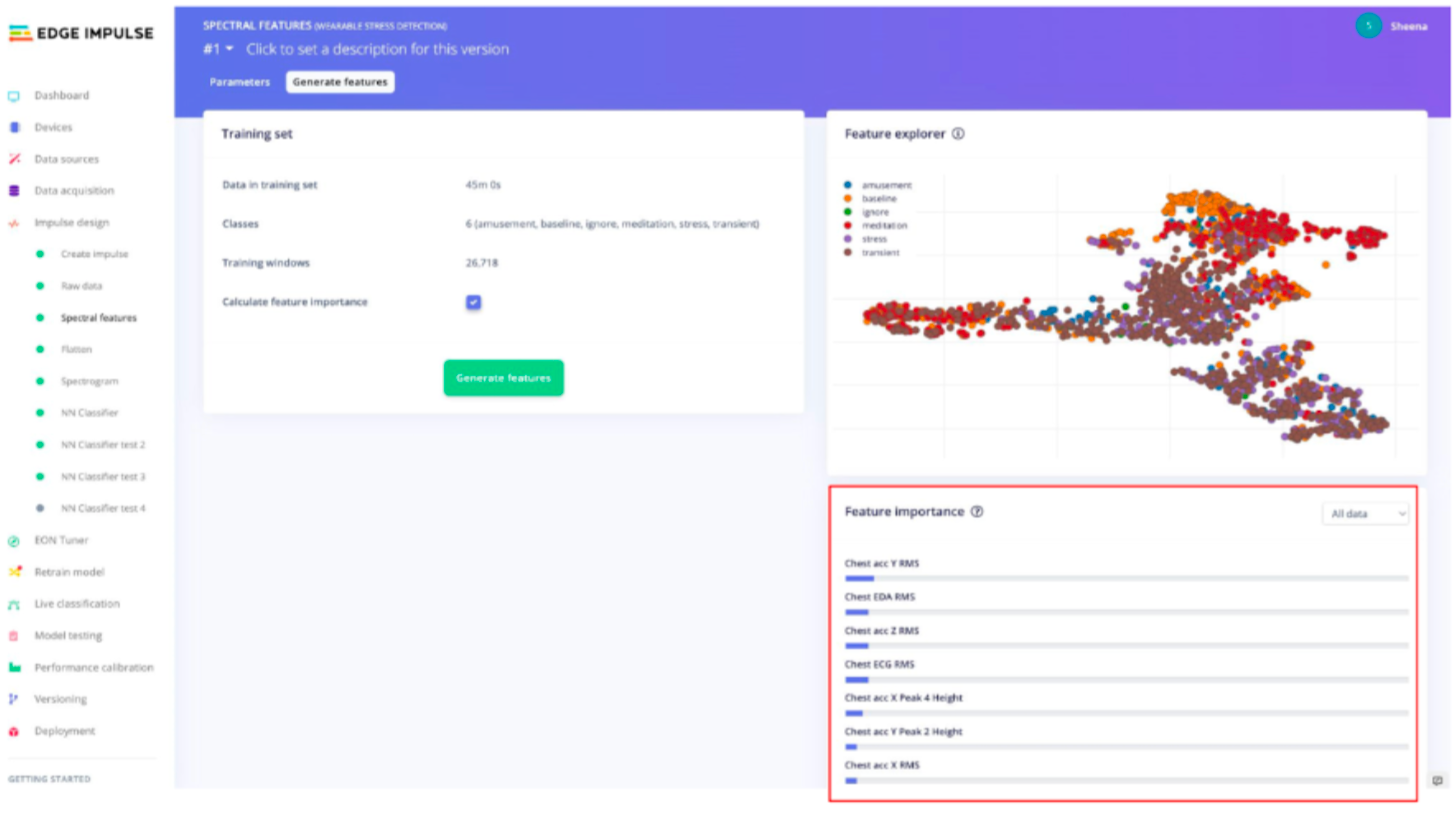
Some use cases Edge Impulse has enabled on devices without the need for cloud compute:
- Predictive heat exhaustion device for firefighters
- Sleep quality analysis and scoring using a wearable ring
- Accurate non-invasive glucose monitoring systems
- Stress monitoring and predictive warning systems
- Fall detection and safety warning devices
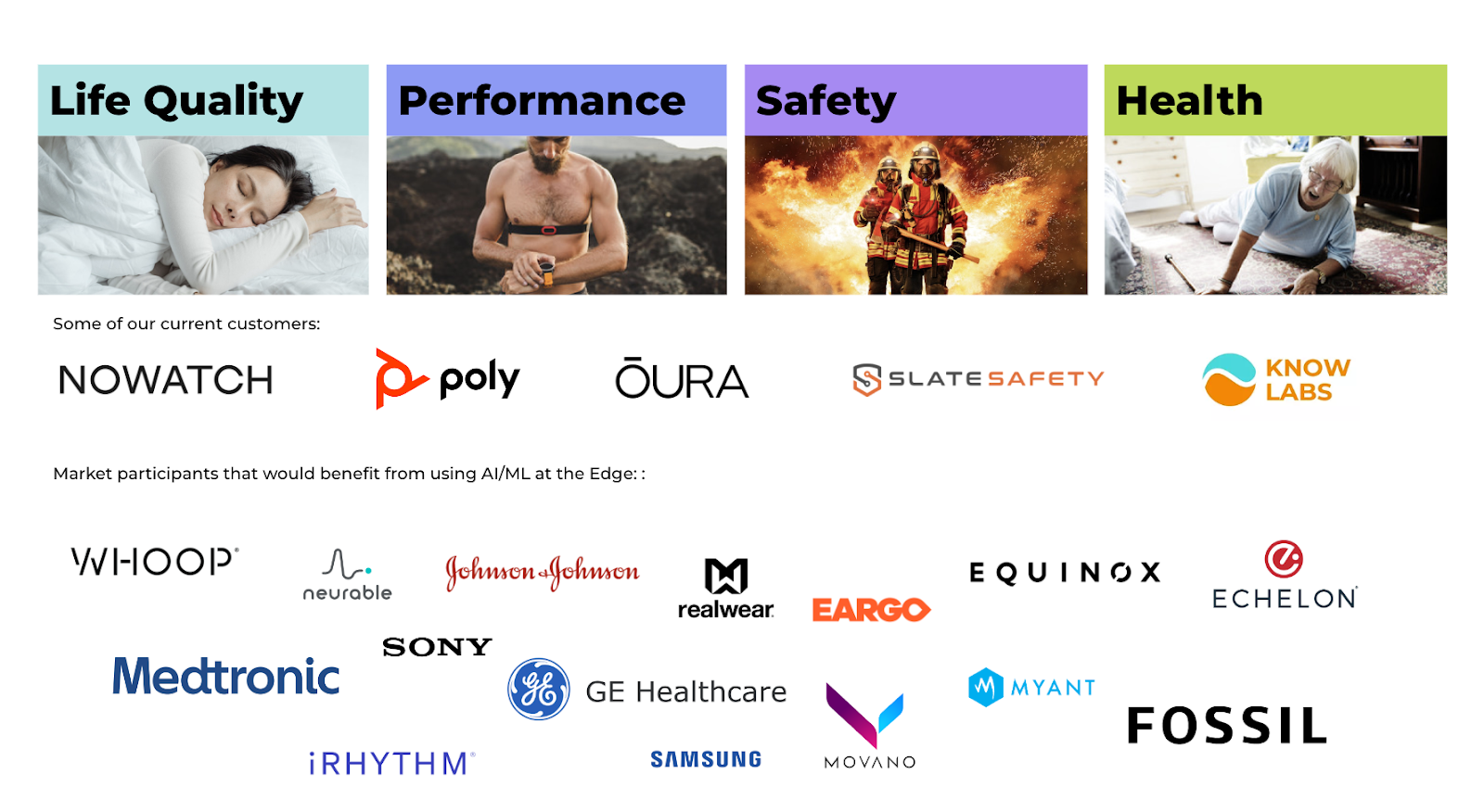
Edge Impulse silicon team
Apart from using the Edge Impulse platform for predictive model creation, Edge Impulse offers a hardware reference design as an infrastructure to scale research studies and product development. Our silicon design team works with our cutting-edge silicon partners and enterprise customers to ensure that the infrastructure is in place to speed up algorithm development time, design with use case computation needs, and decrease go-to-market timing of new health devices.
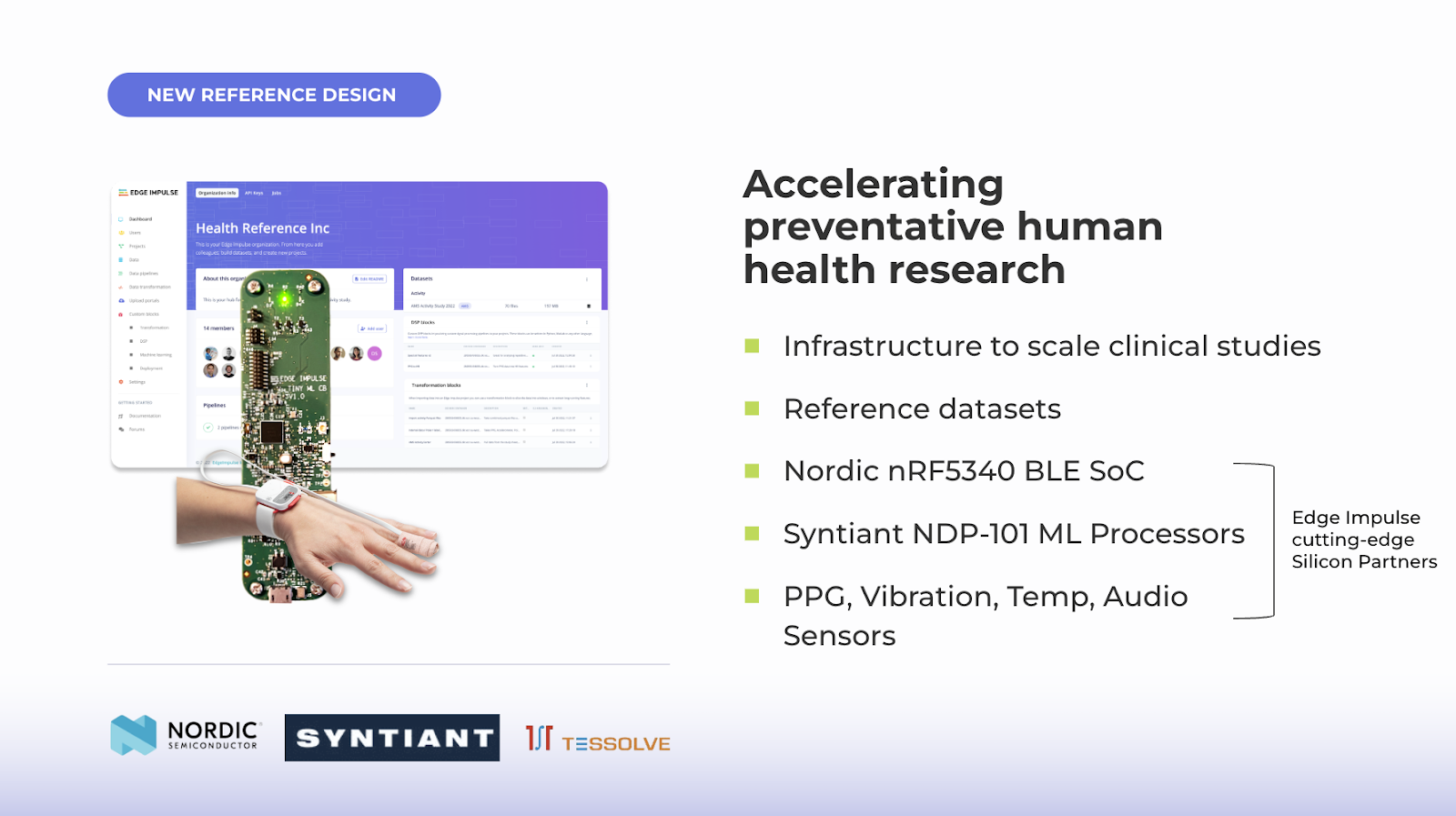
(Watch CEO Zach Shelby introduce our reference design during his Imagine 2022 keynote.)
Talk to our team
With these tools, healthcare developers are able to implement features that offer a competitive advantage while saving time and money. Let’s discuss further on how Edge Impulse enterprise platform can help you bring new human health-related machine learning models, use cases, and devices to market.